Navigating the AI Landscape: Essential Tools for Higher Education Professionals
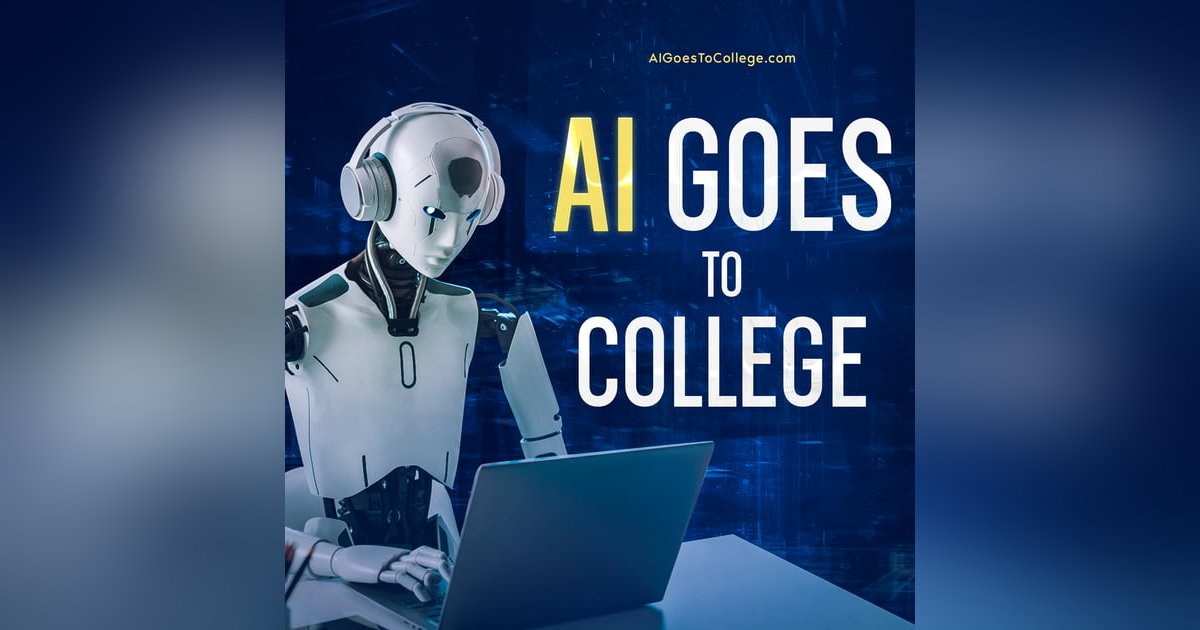
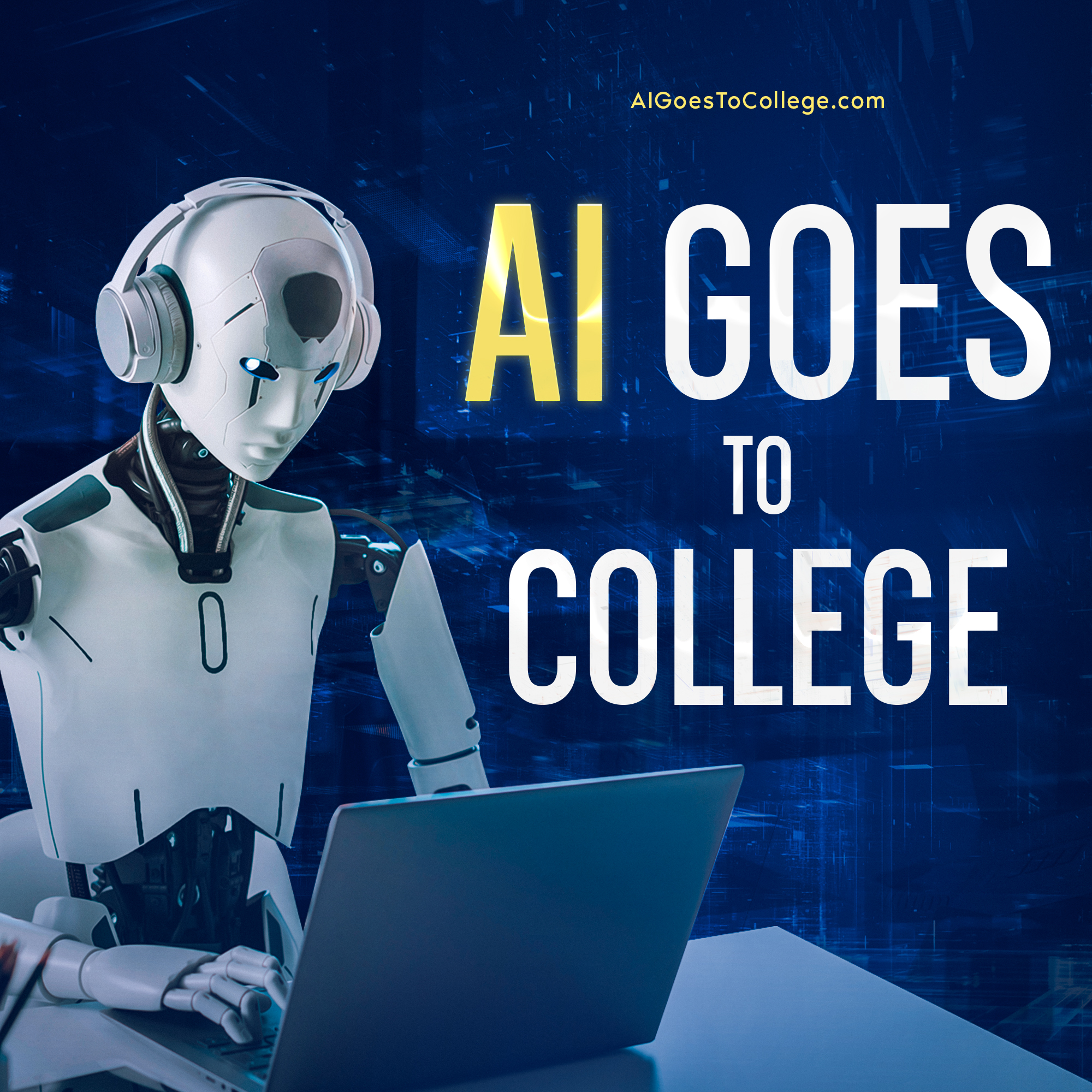
This episode of AI Goes to College discuss the practical applications of generative AI tools in academic research, focusing on how they can enhance the research process for higher education professionals. Hosts Craig Van Slyke and Robert E. Crossler discuss three key tools: Connected Papers, Research Rabbit, and Scite_, highlighting their functionalities and the importance of transparency in their use. They emphasize the need for human oversight in research, cautioning against over-reliance on AI-generated content, as it may lack the critical thought necessary for rigorous academic work. The conversation also touches on the emerging tool NotebookLM, which allows users to query research articles and create study guides, while raising ethical concerns about data usage and bias in AI outputs. Ultimately, Craig and Rob encourage listeners to explore these tools thoughtfully and integrate them into their research practices while maintaining a critical perspective on the information they generate.
---
The integration of generative AI tools into academic research is an evolving topic that Craig and Rob approach with both enthusiasm and caution. Their conversation centers around a recent Brown Bag series at Washington State University, where Rob's doctoral students showcased innovative AI tools designed to assist in academic research. The discussion focuses on three tools in particular: Connected Papers, Research Rabbit, and Scite_. Connected Papers stands out for its transparency, utilizing data from Semantic Scholar to create a visual map of related research, which aids users in finding relevant literature. This tool allows researchers to gauge the interconnectedness of papers and prioritize their reading based on citation frequency and relevance.
In contrast, Research Rabbit's lack of clarity regarding its data sources and the meaning of its visual representations raises significant concerns about its reliability. Rob's critical assessment of Research Rabbit serves as a cautionary tale for researchers who might be tempted to rely solely on AI for literature discovery. He argues that while tools like Research Rabbit can provide useful starting points, they often fall short of the rigorous standards required for academic research. The hosts also discuss Cite, which generates literature reviews based on user input. Although Cite can save time for researchers, both Craig and Rob emphasize the necessity of critical engagement with the content, warning against over-reliance on AI-generated summaries that may lack depth and nuance.
Throughout the episode, the overarching message is clear: while generative AI can enhance research efficiency, it cannot replace the need for critical thinking and human discernment in the research process. Craig and Rob encourage their listeners to embrace these tools as aides rather than crutches, fostering a mindset of skepticism and inquiry. They underscore the importance of maintaining academic integrity in the face of rapidly advancing technology, reminding researchers that their insights and interpretations are invaluable in shaping the future of scholarship. By the end of the episode, listeners are equipped with practical advice on how to navigate the intersection of AI and research, ensuring that they harness the power of these tools responsibly and effectively.
Takeaways:
- Generative AI tools can help streamline academic research but should not replace critical thinking.
- Connected Papers offers transparency in sourcing research papers, unlike some other tools.
- Students must remain skeptical of AI outputs, ensuring they apply critical thought in research.
- Tools like NotebookLM can assist in summarizing and querying research articles effectively.
- Using AI can eliminate busy work, allowing researchers to focus on adding unique insights.
- Educators need to guide students on how to leverage AI tools responsibly and ethically.
Link to Craig's Notebook LM experiment description:
Links referenced in this episode:
- Notebook LM: notebooklm.google.com
- AI Goes to College: aigostocollege.com
- Google Learn About: learning.google.com
- Connected Papers: https://www.connectedpapers.com/
- Scite_: https://scite.ai/
- Research Rabbit: https://www.researchrabbit.ai/
Mentioned in this episode:
AI Goes to College Newsletter
00:00 - Untitled
00:41 - Untitled
00:42 - Introduction to AI Goes to College
01:05 - Exploring Generative AI Tools for Academic Research
13:45 - Exploring New AI Tools for Research
16:57 - Exploring Ethical Concerns with AI Models
24:03 - Exploring Bias in AI Models
31:33 - Exploring Google's Latest Learning Tool
36:07 - Exploring New Tools for Learning
Welcome to another episode of AI Goes to College, the podcast that helps higher ed professionals navigate the world of generative AI.
Craig
At least that's what we're shooting for.
Craig
As always, I'm joined by my friend and colleague, Dr.
Craig
Robert E.
Craig
Krosler from Washington State University.
Craig
Rob, how's it going today?
Rob Krosler
Going great this morning, Craig.
Rob Krosler
How are you doing?
Craig
Good, good.
Craig
Let's just jump right in.
Craig
So recently, you and some of your colleagues and your doctoral students had a pretty interesting session on generative AI tools for academic research.
Craig
Why don't you tell us about that?
Rob Krosler
Yeah, Craig.
Rob Krosler
So we had the opportunity to host a Brown Bag series, which we do almost every Friday at Washington State University.
Rob Krosler
During this Brown Bag, the students from my doctoral seminar who had been working on investigating various different AI tools and how they can help researchers presented their findings and what you can do with four different tools.
Rob Krosler
And in the first part of this podcast, I'm going to discuss three of them, and then we're going to roll into another one that we've talked about before, and I'll share some of the findings with that.
Rob Krosler
So the tools that were presented at this Brown Bag were connected papers, researchrabbit, and Cite.
Rob Krosler
And I'll talk about Connected Papers and Research Rabbit first, because they both work fairly similarly with connected papers.
Rob Krosler
With Research Rabbit, you give it a paper that you've found that you're interested in knowing more about, and it will find the papers that are related to it, and it'll present you a network.
Rob Krosler
So it'll be this network with nodes, which are circles connected by lines.
Rob Krosler
And the circles have different shadings, the lines have different lengths, all indicating something about the paper.
Rob Krosler
They both work very similarly.
Rob Krosler
I really didn't like Research Rabbit, and I'll talk about why before I talk a little bit more about what connected papers can do.
Rob Krosler
So the example that was shown with Research Rabbit took a paper and showed the network, and the network seemed okay, but they don't tell you what the distance of the lines means.
Rob Krosler
They don't tell you what the size of the circles means.
Rob Krosler
They don't tell you what the different shadings of the circles mean.
Rob Krosler
So there's a lot of information that they're not transparent about what it means.
Rob Krosler
They also don't tell you where those papers come from, where's their database, where's their source of information.
Rob Krosler
All of which gave me some concern.
Rob Krosler
But the thing that gave me the most concern is there's a feature where you can click a button to see the papers that this paper Cited.
Rob Krosler
And the individual paper we were looking at cited 83 papers.
Rob Krosler
And in the software it showed you that it cited one.
Rob Krosler
So there's this huge disconnect between the papers it said it cited and what it actually did, which made it to where I felt like I couldn't trust what I was getting.
Rob Krosler
Might it be useful to get started, to go poke and to do some things?
Rob Krosler
Yes, maybe.
Rob Krosler
But once you've used it or three times, then they want to start charging you money for it.
Rob Krosler
And I just did not see the value there, given the lack of trust I saw there.
Rob Krosler
On the other hand, connected papers was a lot more transparent.
Rob Krosler
They shared that they used Semantic Scholar as their database, where they got the papers from.
Rob Krosler
So I was, you know, I've heard of Semantic Scholar, I'm familiar with Semantic Scholar and it has some reputable backing to it.
Rob Krosler
It's not just somebody's made up archive, it's an archive that spreads a great breadth of potential journals and conferences.
Craig
So tell the listeners what Semantic Scholar is.
Craig
Real briefly, please.
Rob Krosler
So Semantic Scholar is a database of, I think it's about 2 million papers that have been published and it brings them, it aggregates them, much like Google Scholar might, but it does it in a different way than Google Scholar.
Rob Krosler
So it's a broad breadth that a lot of databases will rely upon for the appropriate journals to be searching for when you're looking for research.
Rob Krosler
So I did appreciate that.
Rob Krosler
Then it also defined that the distance between the circles or the nodes shows how closely related different ones are.
Rob Krosler
So the further away, the less related it was, the closer together, the more related it was.
Rob Krosler
So if you wanted something that was very closely related, you could dig into those papers.
Rob Krosler
If you wanted something maybe that was further away, you're going to try to bring in some distantly connected stuff that's already been connected with the research stream.
Rob Krosler
You could go look at those papers and then the colors that were listed of the circles actually demonstrated how many times those papers had been cited.
Rob Krosler
So if you saw a connected paper that was a darker color, then you knew that other paper actually had a lot of citations to it, giving you more confidence in how much that paper had been influencing the research and how much it had been used.
Rob Krosler
Was it a one off paper that people really weren't citing yet, or was it one of those seminal papers that a lot of people were citing?
Craig
Do you have any idea how it determined the degree of connection?
Rob Krosler
Yeah, I believe the degree of connection was based on shared citations.
Rob Krosler
So if they had more shared citations between them, they were more closely connected.
Rob Krosler
If they had fewer shared citations, then they were less connected, which I think is useful information.
Rob Krosler
And the other thing that I wasn't sure about though is how it necessarily prioritized what it shared with you is it being all inclusive.
Rob Krosler
And so those papers that maybe were from lesser quality journals just stayed smaller in color and it was inclusive of everything, which is what I think they're doing.
Rob Krosler
But it wasn't 100% clear that it didn't do a level of filtering to bring together.
Rob Krosler
When I look at papers, I'm usually looking for our top tier journals first, our top conferences first, and I didn't necessarily see if it was making any distinguish between that.
Rob Krosler
My hunch is it's not, which I'm okay with because I would rather be the person making that assessment than leaving that up to an algorithm in a machine.
Craig
This gets back to something that's kind of a running theme.
Craig
These tools can help you, but they're not going to do the work for you.
Craig
So they, I think you use something like Jumpstart or get you started earlier and they're good for that.
Craig
They're good for getting a quick handle on an area.
Craig
But I'm with you.
Craig
I wouldn't trust them to make sure it's covered everything I needed to cover.
Craig
So there's still work to be done, but it can, I've been playing with them and they can get you kind of up to speed in an area pretty quickly, which is fantastic.
Rob Krosler
Well, and what I like about it too, and I think where some of the promises is, let's say I did my own search on Google Scholar and I started digging into papers and trying to maybe do forward searches, backward searches, similar theories.
Rob Krosler
However, I might be approaching that I'm likely going to miss something and this is one more tool to maybe help me find a theory that I wouldn't have thought of that's connected and it may open my eyes to something that would actually inform my understanding in ways that I might miss on my own.
Rob Krosler
So I don't think it's a replacement for the way I've been doing searches and the way I've been digging into things.
Rob Krosler
But I think it's a nice addition to know that I've been thorough in trying to identify papers and then it gives it nice access to the abstract so you can read a little bit about what the paper is about very quickly and then a link to go to the paper.
Rob Krosler
And when I'm on my university's network, oftentimes it recognizes I'm on my university's network and it will bring up those papers because our library has access to them and so forth.
Rob Krosler
So it's fairly convenient.
Craig
Yeah, I think there's a little bit of a danger that students might over rely on these tools.
Craig
Not just students, but I think it's more of a danger with students.
Craig
As educators, we're going to have to keep.
Craig
I'm hesitant to use the word hammer, but I think it's the right word.
Craig
We need to keep hammering them.
Craig
Look, these are great tools.
Craig
We want you to use them.
Craig
But ultimately, at the end of the day, your name's on the paper and you've got to be sure that you've done the work that's necessary to find all of the relevant references and bring the right theories to bear.
Craig
And I think that message goes beyond just academic scholarship.
Craig
For those of you who are maybe not on faculty or doctoral students, if you're doing your own research into something that's related to your job, you need to have the same level of human oversight.
Craig
Again, these tools are great for getting a start, for cutting down on a lot of the work that you have to do, but they don't totally do 100% of the work for you.
Rob Krosler
Yeah, Craig and I think that is a nice segue into the third tool that we looked at, which was cite, spelled S C I T E underscore.
Rob Krosler
I think it's pronounced cite, but I'm not certain.
Rob Krosler
And this tool will actually, if you give it a topic, it will write a literature review for you and it will give you paragraph after paragraph with the academic citations.
Rob Krosler
And I don't trust it.
Rob Krosler
But I can see how someone could very easily rely on these tools.
Rob Krosler
And if you didn't know the content, you would think it sounded just fine.
Rob Krosler
As I read the things that it wrote, it sounded plausible.
Rob Krosler
And if I didn't know more about it, I might completely trust it.
Rob Krosler
And it does it actually using academic articles that exist.
Rob Krosler
It did not make up academic articles.
Rob Krosler
It was using papers that are already out there.
Rob Krosler
And so it gave me a lot of concern a of bias.
Rob Krosler
I don't know where it's sourcing its papers from exactly.
Rob Krosler
So I don't know what it is including and what it's not including.
Rob Krosler
Where did it get access to the papers to be able to write a literature review with some level of understanding.
Rob Krosler
But another feature it has is instead of writing the literature review, it will give you a table of the papers and then a summary of what the papers are about related to a given topic.
Rob Krosler
And it'll order them in order of what it thinks is the most significant.
Rob Krosler
I did find that helpful for similar reasons.
Rob Krosler
As I noted about connected papers is it gives you that start of places that you might want to go and look to begin writing your own literature review from your own understanding of the paper.
Rob Krosler
I have a real hard time.
Rob Krosler
And this goes back to that idea of what students might do or what academics might do who want to write about something is I really believe the human element of connecting the dots in your head with what's going on and making your own argument is what is necessary for us to rely on the science that we're creating, as opposed to saying, yeah, a machine wrote all those ideas for me.
Rob Krosler
And I agree with them.
Rob Krosler
Because if you haven't read the papers, if you hadn't made those connections, then it really begins to question what's missing in that human element of the research process.
Craig
What value are you bringing to the process?
Craig
I was just at the College of Charleston giving a couple of talks on AI, and one of the things that I said, it's a message that we need to send to the students pretty strongly, is if all you can do is copy and paste what AI puts out, what does anybody need you for?
Craig
Correct.
Rob Krosler
The value add of what we're being paid for, whether it's as employees or whether it is as researchers or what we're learning is diminished, goes away if we don't bring that critical thought, the human element to these things.
Rob Krosler
It begs the question for why?
Rob Krosler
And I really don't think we're there yet with AI, where it's able to piece together these critical elements like the human mind can.
Rob Krosler
But I do think it's helpful to reveal to the human mind other things we should be considering, other things we should be processing.
Rob Krosler
And in the words of my dean, it's really like having a great intern to where I can go out and do some things for you, gather some things for you, and then help you to be more productive with your time that you didn't necessarily have to spend the hours and hours digging up those papers, but it presents them for you.
Rob Krosler
So you can spend the time that you have to do research that you have for doing your job on the parts where you really do add the value, not where it's busy work going out and gathering at those places you should be looking.
Craig
And that's the message we need to be sending, is you can leverage these tools to cut out the grunt work so that you can focus on where your particular insights and perspectives bring a lot of value to the whether it's to the science or to whatever it is that your job happens to be.
Craig
So I think that's a message we need to keep hammering.
Craig
And I'm sure our listeners will get tired of hearing us say this, but it really is something that we need to be driving home over and over and over again, not only to ourselves, but more importantly to our students.
Rob Krosler
Absolutely.
Rob Krosler
Craig.
Rob Krosler
As someone who teaches a cybersecurity class, one of the things I want all my students to be is skeptical.
Rob Krosler
And I think that absolutely applies in this world of AI is be skeptical of everything and bring your critical thought to all situations.
Rob Krosler
Because the more you begin to just rely on I trust this thing I'm getting, I trust this thing I'm getting, the more likely you are to be being taken advantage of in one way, shape or form.
Craig
Anything else to add on those tools?
Rob Krosler
No.
Rob Krosler
The fourth tool we talked about, and I'm going to use this as a segue to where we're going, was NotebookLM, and as a tool for helping you to answer questions from research articles.
Rob Krosler
You load up these notebooks with papers and then you can start querying them, you can create audio summaries of them, you can create study guides from them.
Rob Krosler
A lot of different things that NotebookLM can help researchers with as they're trying to simplify their understanding of papers.
Craig
Yeah, and We've talked about NotebookLM before.
Craig
I've written about it in the AI Goes to College substack.
Craig
It really is a fantastic resource.
Craig
The real quick 30 second overview is this is a tool from Google.
Craig
It's notebooklm.google.com where you can upload your own resources.
Craig
We're talking about papers, but it could be websites, it could be videos, it could be Google Docs, it can be a lot of different things.
Craig
And then it will use those documents as the foundation for whatever it is that you're trying to accomplish.
Craig
And so it's very cool.
Craig
I think it's among the most interesting AI tools that we've got right now.
Craig
And it is absolutely fantastic for certain kinds of academic research.
Craig
But not only that, you can upload your university's policies around something or your university's recruiting materials or whatever it might be, and use that as the basis for your conversations with NotebookLM.
Craig
What did you all talk about in your seminar?
Rob Krosler
Yeah, well, we talked about basically using it to load papers that you were interested in and using it to query.
Rob Krosler
You could ask it what theories it used, you could ask it what constructs it used.
Rob Krosler
One of the things that was very useful is to create a learning guide from it where we would summarize the document in a way that helps you to pull out some of the key features.
Rob Krosler
Some of the concerns were around whether or not you could completely trust that it did a good job.
Rob Krosler
The evidence provided that maybe it doesn't was when you create the podcast or the visual overview, that sometimes it would ignore certain papers and putting those things together.
Rob Krosler
Is that happening when you have too large of a notebook, when you're trying to get this more written summaries?
Rob Krosler
And then another concern that was brought up that I'm not certain I know the answer to, and maybe you can shed some light on this, Craig, is does Google train off of that information?
Rob Krosler
So if you upload, say, a paper that you have written and you're asking it to help you do some things with your own stuff that hasn't been published yet, are you functionally giving that away to Google to train their models to do their things?
Rob Krosler
No one had a perfect answer for that when we were discussing that.
Rob Krosler
And that raised some concerns as well, I would assume.
Craig
Yes, because it probably is.
Craig
I don't know the answer to that question, but I think I would behave under the assumption that it is, although I'm not as worried about that as a lot of other people are.
Craig
I mean, I just think the probability of somebody scooping my idea based on how the model gets trained is pretty low.
Craig
When you think about it, it's got to be trained and then it's got to be tested and then it's got to be rolled out and then the query has to be such.
Craig
So I don't know, Greg, where I.
Rob Krosler
Think the interesting thing is though, is we're pretty quick perhaps to upload copyrighted content, and if you don't have permission to upload that copyright content to query and then you're giving it away, are you breaking the law or operating unethically?
Rob Krosler
So I think there is some interesting conversations to have around the ethical nature of how all of these models are being trained.
Craig
I was talking about your own work, but you absolutely shouldn't upload something that's copyrighted without permission.
Craig
I think that's morally wrong to begin with.
Craig
Yeah, that's a bad idea.
Craig
So I would agree with you there.
Craig
There's another problem that may or may not have come up in your conversation, but I've had it come up about NotebookLM, and that's its apparent bias.
Craig
So the quick backstory.
Craig
I was at the POD Indie Conference, which is an annual conference in Indianapolis for podcasters.
Craig
And I was co headlining with Dave Jackson, who's a very well known podcaster and podcast coach.
Craig
I was doing AI stuff.
Craig
My big finish was Notebook lm.
Craig
And so I had taken a bunch of transcripts from my personal podcast, Live well and Flourish, all about stress and stress management, put it into Notebook lm.
Craig
And then, Rob, you mentioned the audio overview, which is kind of an NPR style podcast that it produces, which frankly is just this side of magic.
Craig
It's so good.
Craig
And so I did that, played it, and two of the people at the conference said, is there any way to make it so the woman doesn't sound like an idiot?
Craig
And that was coming from a female.
Craig
Two females, actually.
Craig
And I got to thinking and it does seem to make the female character.
Craig
So there's a male character and a female character, a male sounding, female sounding character in the audio overview.
Craig
And the male does seem to drive the conversation.
Craig
That's been my experience.
Craig
I wasn't so sure I would have said the female sounds like an idiot.
Craig
Rob, have you experienced the same thing when you've used it?
Rob Krosler
I have, and it's very interesting.
Rob Krosler
And this may show my bias is I didn't pick up on it until you said something.
Rob Krosler
And once it was pointed out, I was like, yeah, absolutely.
Rob Krosler
It's very much driven by the most sounding host in that podcast.
Rob Krosler
So I was really intrigued by the little research you did to poke into this.
Craig
The first thing I did was try the custom instructions.
Craig
So it has some kind of custom instructions where you can tell it to focus on certain aspects of your resources that you uploaded.
Craig
So I put in there, have the female lead the conversation.
Craig
I don't think it did anything at all.
Craig
It was worth a try.
Craig
It didn't seem to work.
Craig
So what I did is I wanted, you know, we're scientists.
Craig
So I wanted to do an experiment.
Craig
So I took six different resource sets.
Craig
So I set up six different notebooks in Notebook lm, I had it do the audio overview.
Craig
And then I transcribed each one of those audio overviews using Otter AI, which is fantastic for this kind of thing.
Craig
Then took those transcripts, put them in ChatGPT, Gemini and Claude and asked three questions, said, you know what?
Craig
One of these is a male sounding character, which I labeled, and then a female sounding character, which I also labeled with different names, Sheila and Kyle.
Craig
For the female and male, that's a shout out to Dave Jackson.
Craig
Those are the names he likes to use.
Craig
And I said, which one led the conversation?
Craig
Which one dominated the conversation and which one Sounded smarter.
Craig
And it had three choices, Sheila, Kyle, or neither.
Craig
So I know that's a lot of information and I'll put this in the show notes.
Craig
So, in terms of leading the conversation, Kyle led the conversation 67% of the time, or in 67% of the experiments.
Craig
So remember, we've got six conversations, three different analyses for the different chatbots.
Craig
So we've got 18 trials here.
Craig
I think it's 18.
Craig
Did I do that math right?
Craig
Anyway, 67% of the time, Kyle came out as leading the conversation, 27% of the time, it was Sheila who dominated the conversation.
Craig
It was pretty similar.
Craig
60% of the time it was Kyle, and 33% of the time, it was Sheila.
Craig
So we've got a trend going here where the male character is definitely driving the bus.
Craig
But then which one sounded smarter?
Craig
Kyle came up as sounding smarter 0% of the time, which I thought was pretty interesting.
Craig
60% of the time, the AI chatbot said that neither one came off as smarter.
Craig
And then 40% of the time, it was Sheila that sounded smarter.
Craig
I thought this was pretty interesting and pretty curious.
Craig
So I don't know.
Craig
I'm going to pause here, ask you for your reactions.
Rob Krosler
My first reaction is, how is smarter determined?
Rob Krosler
I honestly don't know what determines that.
Rob Krosler
The other parts of it aren't terribly surprising, based on the times I've listened to it, that it was dominated or led by the male sounding voice or by Kyle, but one being smarter than the other.
Rob Krosler
Again, to me, that seems like the tools are introducing their bias on determining what smart means.
Craig
Well, it's funny you should say that, because I ask it to explain its reasoning for each one of these assessments.
Craig
And with smarter, it was adding more concrete details, giving more examples, having more breadth than the answers, those sorts of things.
Craig
And if anybody's interested, you can email me@craigaigostocollege.com and I will share the complete transcripts of the chat sessions with you.
Craig
But that's essentially what they did.
Craig
If there were more details, if there was more concreteness, more precision in the comments, then that's what it tended to think as being smarter, which, I don't know.
Craig
That kind of made sense to me.
Craig
So what was more interesting?
Craig
Well, not more interesting, but what was also interesting is I dug into why that might be exhibiting bias, and I thought, this needs more investigation.
Craig
This all needs more investigation.
Craig
But I thought.
Craig
I thought this was fascinating.
Craig
So we have gender stereotypes where males tend to lead things and dominate things, but the females are the people that we might draw on.
Craig
For certain kinds of expertise.
Craig
And so that's kind of what the models were saying was the basis for calling this biased.
Craig
So all three of the models thought these were biased results, that these results exhibited bias.
Craig
So anyway, I think there's a lot more research that needs to be done in this, but I think there's definitely something going on here.
Rob Krosler
Yeah, Craig, it'll be interesting to see how Notebook LM advances as well.
Rob Krosler
I've read some things that suggest that there could be other approaches they use for these audio summaries that might be different than this whole NPR podcast style.
Rob Krosler
And will there be the abilities to pick and choose the types of voices, or will it be more being able to write audiobooks and various different things?
Rob Krosler
It'll be interesting how this bias plays a role in this various other different ways that we're going to have these audio overviews as well.
Craig
I think there's a high level issue that we want to make sure that we cover.
Craig
There's bias of many different kinds in the data that these models, these large language models that drive the chatbots, are trained on.
Craig
And that's just a fact.
Craig
There is bias in those data sets.
Craig
And unless we do something to mitigate that bias, that bias is going to creep into the outputs that the large language models, including the chatbots, give us on the surface.
Craig
Okay, well, let's do something to mitigate those.
Craig
But how do you mitigate those?
Craig
So you kind of introduce your own bias in how you mitigate.
Craig
So it's kind of a mess.
Craig
And I'm not sure what we do about it beyond going back to one of our running themes, making sure that you go through the output that you generate with the help of generative AI and make sure that you aren't inadvertently perpetuating some kind of bias.
Craig
And this is not woke stuff here.
Craig
I mean, this is just fact that these biases are in there and that those biases can create harm for people.
Rob Krosler
Yeah, absolutely.
Rob Krosler
I think owning the material, being transparent in how you've used it, and taking responsibility for what you create and doing the legwork so you can take that responsibility.
Rob Krosler
An important, just default behavior that you should have with these different tools.
Craig
Yeah, just like anything that you're putting out into the world, you know, you don't want to put things out that cause harm inadvertently or any other way.
Craig
All right, anything else on bias?
Craig
I'm sure we'll return to this at some point and I'm really thinking about doing a much more systematic investigation of this because one of the reasons is that Your students, whether you know it or not.
Craig
And this is not just for you, but any of our listeners out there that teach your students are using Notebook LM to help them study.
Craig
Absolutely.
Craig
What are the long term implications of that?
Craig
And I don't blame them.
Craig
I would, too.
Craig
I think it's brilliant.
Rob Krosler
I encourage my students to use it, but I encourage them with the whole idea that they also need to do more than just that if they want to truly understand what's going on.
Rob Krosler
But I think it changes the way we teach in the classroom, too, where we need to engage more than just here's what the textbook said and here's me regurgitating what the textbook said.
Craig
Maybe we should just do NPR style lectures.
Craig
So, Rob, today we're going to talk about privacy and information systems, you know, whatever it might be.
Craig
Okay.
Craig
The caffeine's kicking in, so we should probably move on.
Craig
All right, so I did want to talk about two other tools that I think are pretty significant.
Craig
One is ChatGPT Search, which has been out for, I don't know, a couple of months now.
Craig
And if you use ChatGPT, if you look down at the text box where you put your prompt in, you'll see a little globe.
Craig
And if you click on that globe, you're basically telling ChatGPT to go out and search the web when it's developing its answer.
Craig
Have you used that much yet?
Rob Krosler
I've used it once or twice and found it useful.
Rob Krosler
But I'll tell you, my struggle is Google has Gemini, ChatGPT has their search, and everybody has these different searches.
Rob Krosler
And when one seems to be working fairly well for me, what's the cost of changing?
Craig
Yeah, that makes sense to me.
Craig
Although where I might disagree is Gemini is not quite as good at some things as ChatGPT is.
Craig
And so if you're doing a quick thing, that would be like a web search.
Craig
We've talked about this before.
Craig
Gemini is fantastic.
Craig
But if you want to go further than that, sometimes I find Gemini a little bit limiting.
Craig
That's when I turn to ChatGPT search, because you still get all the things that ChatGPT is good at, at least most of those things while you're doing search.
Craig
The downside is you, at least last time I looked, you could not attach documents and do search in the same prompt, which is a little bit limiting if it's something that's extensive.
Craig
Perplexity works really well for me.
Craig
So I'm a big fan of perplexity AI for anything where I wanted to go out and search the web or look at particular documents.
Craig
So we'll see.
Craig
I mean, hey, if it works well for you, okay, if it doesn't, okay, don't use it.
Craig
Then it's kind of my attitude.
Craig
But for the listeners who haven't played with it, I think it's worth playing with.
Craig
And just to be really clear, you can turn it on and off within a conversation, but you can't search and upload a document in the same prompt.
Craig
Rob, Anything else on ChatGPT search?
Rob Krosler
Well, the only thing I would add, and this is, I think the struggle that people are going to have to ultimately go down the pathway is are you going to be the person who uses all of these tools?
Rob Krosler
Are you going to be like Craig, or are you going to explore a little bit and decide what is your perfect set of tools?
Rob Krosler
Because at the end of the day, A, whether it's the number of subscriptions you're willing to pay for, or B, whether it's the cognitive memory of which tool do I need to go to when you're going to come to a point where you say, this is good enough for what I want to achieve?
Rob Krosler
So I would encourage everyone to play with these and to come up with your own assessment of what's useful for how you seek out information and seek out answers to things.
Craig
Yeah, absolutely.
Craig
Don't be like Craig, because, I mean, I just like fooling around with this stuff.
Craig
So, yeah, that's probably not a productive way to do this.
Craig
Although I think when you hear about a new tool, you might want to check it out because you may find that it's better than what you're using.
Craig
And virtually all of these tools have a free tier, which is fine for most uses, so you don't have to spend a lot of money to do this.
Craig
All right, speaking of new things Google learn about and it's learning L E A R N I n g google.com and that's Google's Learn about.
Craig
This is a really cool new tool.
Craig
So basically you go to learning.google.com type in something that you want to learn about.
Craig
How do large language models work, hit Enter, and it kind of creates a mini textbook chapter on that topic, including pointing out to websites where it pulled the information from that sort of thing.
Craig
You can ask for more details.
Craig
You can go down different branches.
Craig
It's really cool.
Craig
Have you had a chance to play with it yet?
Rob Krosler
Yeah, I played with it.
Rob Krosler
I was impressed.
Rob Krosler
And as someone who's a textbook author, at some point I start to wonder, at what point are textbooks Irrelevant, because you're going to be able to dynamically create exactly what you want on the fly and guide your students that way, as opposed to saying we're all going to use the same exact textbook.
Rob Krosler
It was scary.
Craig
Yeah, it is.
Craig
I think the problem with it right now, there are a couple of problems.
Craig
One is it's not curated.
Craig
So you would put a lot of effort into curating the material and organizing it and thinking about the flow of the course and that sort of thing.
Craig
So that's a big reason we use textbooks, is because it's a way to organize everything really easily.
Craig
But who knows in 20 years or 10 years.
Craig
Yeah.
Rob Krosler
Well, I see especially with young faculty, they really rely heavily on textbooks for what they want to do.
Rob Krosler
For that exact reason.
Rob Krosler
My more mature faculty have been doing this for 20, 20 plus years.
Rob Krosler
They're more apt to feel like I kind of know the path, the direction I want to go.
Rob Krosler
And so is the value going to be on mentoring young faculty on how to utilize these tools to curate and create a well designed course as opposed to asking our students to spend $150, $200 on a textbook to go through that process for them?
Rob Krosler
So it's going to be really interesting to see how that process plays out.
Craig
Or they can just use a textbook that doesn't cost that much like ours.
Rob Krosler
Amen.
Craig
It's going to put pressure on the textbook publishers and I think that's a good thing.
Craig
Overall, there's a huge problem with it right now though.
Craig
Once you close out that window, that session is gone forever.
Craig
So right now it cannot save sessions, which is a big drawback for instructional use.
Craig
You can just save the, save it as a webpage and that seems to work reasonably well.
Craig
But you can't go back in and say, oh, I should have asked it this question, it's just gone.
Craig
But my guess is that'll get solved eventually.
Craig
But where?
Craig
I think this is maybe a little bit of a watershed moment.
Craig
Something that higher ed has been pushing, particularly in our field, information systems, for as long as I've been around, is we need to help students become self directed, lifelong learners.
Craig
We're not so great at explaining exactly how they should do that, but we do push that, especially in a dynamic field like information systems.
Craig
This could be a big step in that direction and maybe even more importantly to democratizing learning.
Craig
So where you don't have to pay somebody, you don't even have to sit at the feet of the masters to learn things, you can learn it on your own.
Craig
And that might be bad for higher ed, but I think not if we do things correctly.
Craig
But it really is good for learning.
Craig
So I think that's why this learn about.
Craig
And I'm sure there will be other tools along these same lines.
Craig
I think that's where this is.
Craig
This is really, really important.
Rob Krosler
Yeah, absolutely.
Rob Krosler
I think, you know, you've probably experienced with your students as well, is they come up to you after class and they want to go deeper onto a topic.
Rob Krosler
They want to know where else they can go.
Rob Krosler
And I can see this absolutely.
Rob Krosler
Engaging those who are becoming curious about the topics and wanting to engage with them further beyond where the classroom activities may have taken them.
Craig
Yeah.
Craig
And this could be a great assignment.
Craig
Just here's your topic.
Craig
Create a learn about.
Craig
Or you could do the same kind of thing with perplexity.
Craig
Create a perplexity page.
Craig
That's not easy to say perplexity page about this topic.
Craig
So I'm really excited about these tools.
Craig
I know a lot of our colleagues at higher ed are justifiably feeling a little bit overwhelmed right now with all of this.
Craig
It's going to settle out over the next five or six years, I think, and we're here to help you.
Craig
So one of the best, and this is a plug, but I really mean this.
Craig
One of the best things you can do is listen to podcasts like this, read newsletters like AI Goes to College, and help us sift through some of these things where you can direct your energies, where they're going to do the most good.
Craig
All right, we've covered a lot of ground here.
Craig
Any last thoughts, Rob, before we close?
Rob Krosler
Yeah, two thoughts.
Rob Krosler
One, just a reminder, play with the tools, get to know them, get to figure out how you would use them, and settle on the ones that you like.
Rob Krosler
Another plug I'll make is if there's something you want Craig and I to talk about that we haven't touched on, please let us know and we can dig into it and we can potentially bring it in as a topic.
Rob Krosler
And maybe, if Craig's willing, maybe we'll have guest visitors who might be able to shed some light from a different perspective along the way.
Craig
Yep.
Craig
And along those lines, you can go to aigostocollege.com and there's a little contact us button.
Craig
I think it's in the lower right that you can use to contact us or you can email me@craigaigoestocollege.com One of the things that Rob and I have talked about is hosting an Ask Us Anything session.
Craig
So if that's something you'd be interested in.
Craig
Send me an email, use the contact us@aigostocollege.com and let us know if that's something.
Craig
You should see the look on Rob's face.
Craig
He's like, what you did.
Craig
We talked about this.
Craig
So I think it'd be maybe a useful thing for people.
Craig
All right, two other real quick things.
Craig
If you want either one of us to come to your campus, either virtually or live, and talk to your faculty, talk to your staff, your colleagues, your students, let us know.
Craig
Talked about how you can contact us.
Craig
We are both willing to do that.
Craig
We do that sort of thing on a regular basis and we'd love to help you out.
Craig
And then the last thing is, I have, I talked about Lex.
Craig
Lex Page is a AI enabled low distraction writing environment which I use all the time.
Craig
Now they reached out to me and they are offering a 25% discount on the annual pro plan if you use the discount code aigtc.
Craig
We don't get anything out of this.
Craig
It's not an affiliate deal.
Craig
It's just something that they wanted to do for AI Goes to College listeners.
Craig
If you haven't used it, check it out.
Craig
The free version is actually quite good.
Craig
It's like the copy editor that you wish you had on call 24 7.
Craig
It's really a fantastic tool, so I encourage you to check it out.
Craig
25% off using the discount code AIGTC.
Craig
All right, I'm tired.
Craig
Anything else, Rob?
Craig
All right, that's it for this time.
Craig
We will see you next time on AI Goes to College.